RGHGF Interview Series: Dr. Ning Wen at Henry Ford Health System
- RGHGF Staff
- May 3, 2020
- 4 min read
In the second installment of RGHGF’s Research Interview Series, the team sat down with Dr. Ning Wen of Henry Fold Health System (HFHS). Dr. Wen’s work at HFHS on deep machine learning algorithms to develop biomarkers which aid in prostate cancer (PCa) risk classification and subsequent therapy is carving a niche in the field of PCa research. We asked him a number of questions regarding his background in science, research motivations, and his views on the landscape of cancer research.
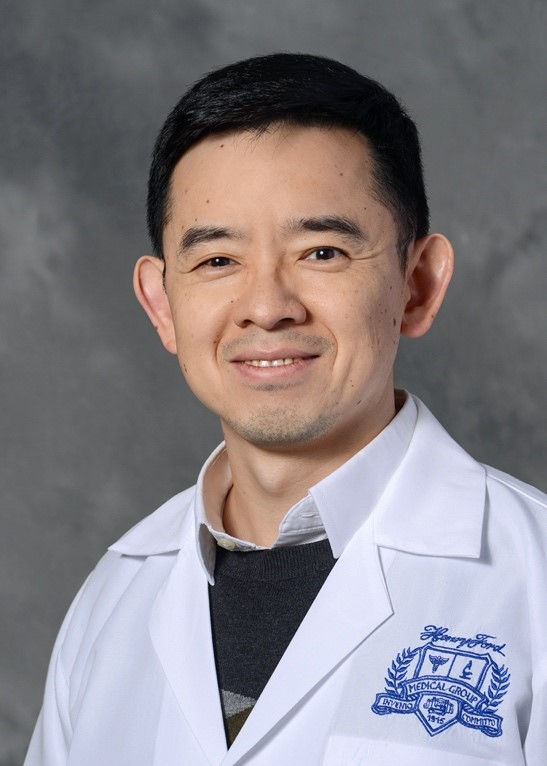
Pictured: Dr. Ning Wen, Ph.D., Director of Clinical Physics at Henry Ford Health System
RGHGF: Could you tell us a little bit about your personal background?
Dr. Wen: I finished my Ph.D. degree at Wayne State University and have been working at Henry Ford Health System since 2007. My interests are in many aspects of professionalism in medical physics, including clinical care, research, and education. I serve as the Director of Clinical Physics at Henry Ford Health System and have published more than 70 peer-reviewed papers. I have been serving as a principal investigator on a Research Scholar Grant from the American Cancer Society and a co-investigator on several NIH or industry funded research grants.
RGHGF: How did you become interested in cancer and radiology research over other forms of scientific research?
Dr. Wen: I think cancer research found me; I never thought I would pursue a career in cancer research. It blew my mind when I learned advanced imaging and therapeutic development in radiological physics and decided to pursue an advanced degree in medical physics.Medical physics is a unique and multidisciplinary field integrating physics principles, mathematical modeling, and biological frameworks into problem solving. I had the opportunity to pursue my PhD in medical physics and develop my passion to innovate and implement advanced technology to transform cancer treatment.After working in a clinical environment for cancer care, I became enthusiastic about molecular biology and decided to take an integrated approach to work on cancer research.
RGHGF: Please tell our readers about a unique research program or project at the HFHS.
Dr. Wen: With wide-spread (prostate-specific antigen) PSA screening, the overall age-adjusted incidence of prostate cancer has increased gradually since 1986. The combination of PSA, clinical stage, and Gleason score have also increased the detection of prostate cancers (PCa) at earlier stage. The systematic radical approaches, including surgery and radiation, have been the gold standard for prostate cancer treatment and very effective at controlling low-risk, localized diseases. However, dose escalation could always increase potential treatment toxicity and induce diminished quality of life outcomes. A survey in the Netherlands even showed that 75% of patients would choose to be treated with a lower dose for a lower cure rate to avoid toxicity.
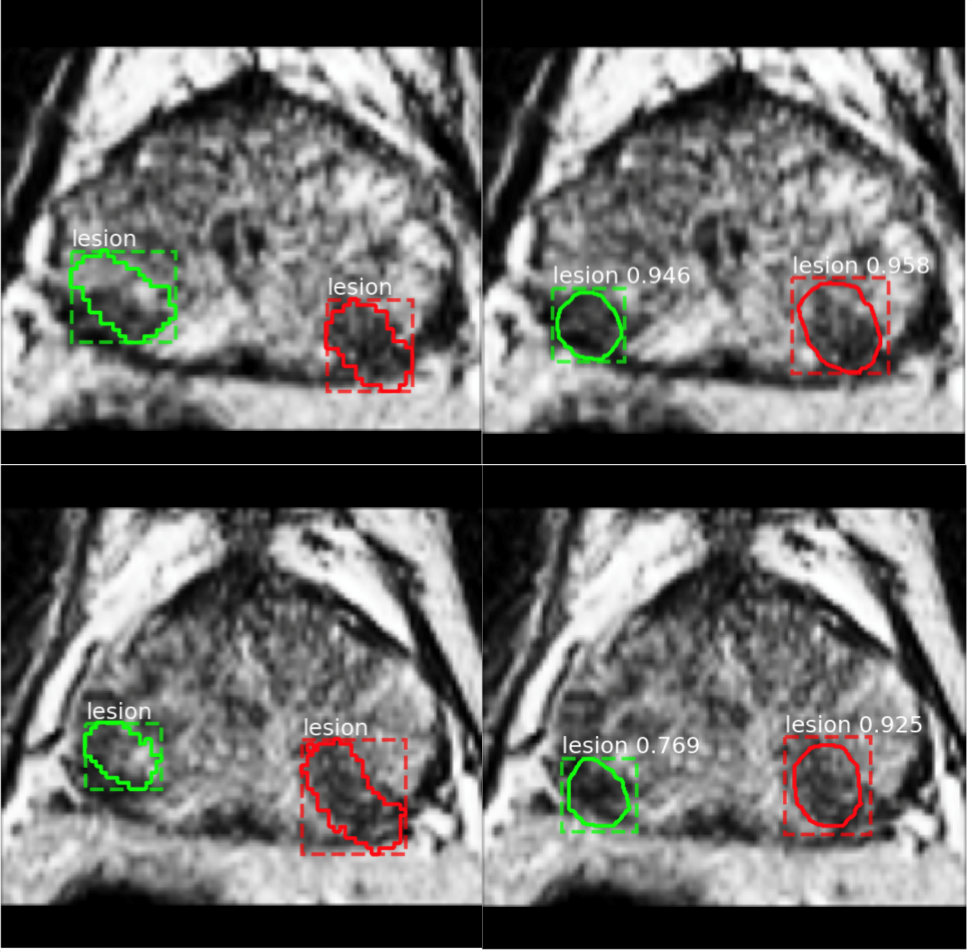
Lesion segmentation on two continuous slices from one patient, with two lesions identified and contoured (green and red) on T2WI. Ground truth by the clinician (left column) is shown with lesion contour and bounding box; prediction of two agreed candidate lesions by the deep learning algorithm (right column) is shown with the bounding box, lesion contour as well as prediction class and score.
We aim to develop deep machine learning algorithms to identify imaging and molecular biomarkers that will facilitate accurate PCa risk classification and the development of corresponding precision radiotherapy. Newly discovered PCa genetic signatures with an underlying spectrum of molecular abnormalities have been valuable in predicting tumor progression and metastasis. In addition, PCa physiological and phenotypic heterogeneities can also be deduced using quantitative imaging features derived from multiparametric magnetic resonance imaging (mpMRI). Hence, integrating genetic biomarkers with phenotypic mpMRI features in a multiplex setting has an enormous potential to identify PCa biomarkers crucial for: 1) improving patient risk stratification, 2) developing precision RT to escalate dose to intraprostatic lesions, and 3) predicting RT response.
RGHGF: Do you have any collaborations with other cancer researchers or scientists in different disciplines? How have these interactions shaped your professional interests?
Dr. Wen: I have been collaborating closely with researchers and clinicians in other specialties. The Henry Ford Cancer Center offers a very good platform for researchers and clinicians to work closely on cancer research. We need a multidisciplinary approach to develop machine learning algorithms to identify imaging biomarkers and genomic biomarkers that have diagnostic and prognostic values. To a man with a hammer, everything looks like a nail, as an old saying said. I was trained as a physicist. Working together with radiologists, radiation oncologists, MR scientists, pathologists, urologists and computer scientists, I am able to think more deeply, differently and broadly on the research topics.
RGHGF: What excites you about the future of cancer treatment?
Dr. Wen: In the precision medicine era, with the huge omics data taken from medical images, cancer genome and breakthrough of deep machine learning in data analytics, I have seen great opportunity to address cancer treatment in a data driven approach. This leads to the future exploration to conquer treatment and improve patients’ quality of life in a multidisciplinary approach with the collaborations from experts in medicine, physics, biology, computer science, engineering, etc.
Thanks to Dr. Wen for this fantastic interview and for sharing your journey in cancer research!
Yorumlar